Adversarial Robustness of Compressed Models
Create and release your Profile on Zintellect – Postdoctoral applicants must create an account and complete a profile in the on-line application system. Please note: your resume/CV may not exceed 3 pages.
Complete your application – Enter the rest of the information required for the IC Postdoc Program Research Opportunity. The application itself contains detailed instructions for each one of these components: availability, citizenship, transcripts, dissertation abstract, publication and presentation plan, and information about your Research Advisor co-applicant.
Additional information about the IC Postdoctoral Research Fellowship Program is available on the program website located at: https://orise.orau.gov/icpostdoc/index.html.
If you have questions, send an email to ICPostdoc@orau.org. Please include the reference code for this opportunity in your email.
Research Topic Description, including Problem Statement:
Machine learning models, particularly deep neural networks, have shown remarkable performance across a range of domains. However, their vulnerability to adversarial attacks poses significant security concerns. This research aims to explore the relationship between two model compression techniques – distillation and quantization – and their impact on the robustness of ML models against adversarial attacks. Previous studies have shown that distillation and quantization may improve model robustness through transfer of knowledge from robustly trained teacher models [1] and through the introduction of noise to mitigate adversarial perturbations [2]. Other studies have shown that the relationship between adversarial robustness and quantization is more nuanced and depends on the strength of the attack [3].
This research will evaluate the robustness of distilled and quantized models against a range of adversarial attacks, investigate the combined effect of quantization and distillation on model robustness and examine the factors that affect robustness when compressing models.
Example Approaches:
Possible approaches are:
- testing models distilled using robustly trained teacher models against common adversarial techniques.
- applying quantization to robust models and assess their performance against common adversarial techniques
- evaluating and developing distillation procedures that improve model robustness.
- analyzing which properties of deep neural networks (architecture, activation functions, loss functions etc.) play significant roles when assessing the adversarial robustness of compressed models.
Relevance to the Intelligence Community:
This research will contribute to the development of more secure and efficient ML models which is crucial for deploying models in real-world situations where security is paramount.
References:
- [1] Zi, B., Zhao, S., Ma, X. and Jiang, Y.G., 2021. Revisiting adversarial robustness distillation: Robust soft labels make student better. In Proceedings of the IEEE/CVF International Conference on Computer Vision (pp. 16443-16452).
- [2] Ayaz, F., Zakariyya, I., Cano, J., Keoh, S.L., Singer, J., Pau, D. and Kharbouche-Harrari, M., 2023, June. Improving Robustness Against Adversarial Attacks with Deeply Quantized Neural Networks. In 2023 International Joint Conference on Neural Networks (IJCNN) (pp. 1-8). IEEE.
- [3] Gorsline, M., Smith, J.T., & Merkel, C.E. 2021. On the Adversarial Robustness of Quantized Neural Networks. Proceedings of the 2021 Great Lakes Symposium on VLSI.
Key Words: Adversarial attacks, machine learning models, distillation, quantization.
Postdoc Eligibility
- U.S. citizens only
- Ph.D. in a relevant field must be completed before beginning the appointment and within five years of the appointment start date
- Proposal must be associated with an accredited U.S. university, college, or U.S. government laboratory
- Eligible candidates may only receive one award from the IC Postdoctoral Research Fellowship Program
Research Advisor Eligibility
- Must be an employee of an accredited U.S. university, college or U.S. government laboratory
- Are not required to be U.S. citizens
- Citizenship: U.S. Citizen Only
- Degree: Doctoral Degree.
-
Discipline(s):
- Chemistry and Materials Sciences (12 )
- Communications and Graphics Design (3 )
- Computer, Information, and Data Sciences (17 )
- Earth and Geosciences (21 )
- Engineering (27 )
- Environmental and Marine Sciences (14 )
- Life Health and Medical Sciences (45 )
- Mathematics and Statistics (11 )
- Other Non-Science & Engineering (2 )
- Physics (16 )
- Science & Engineering-related (1 )
- Social and Behavioral Sciences (30 )


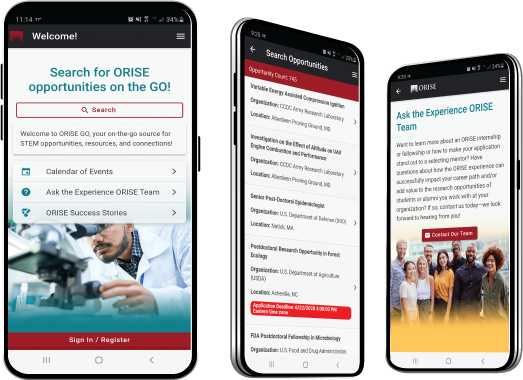
The ORISE GO mobile app helps you stay engaged, connected and informed during your ORISE experience – from application, to offer, through your appointment and even as an ORISE alum!