Utility of Synthetically Generated Data for Training or Testing AI/ML Systems
Create and release your Profile on Zintellect – Postdoctoral applicants must create an account and complete a profile in the on-line application system. Please note: your resume/CV may not exceed 3 pages.
Complete your application – Enter the rest of the information required for the IC Postdoc Program Research Opportunity. The application itself contains detailed instructions for each one of these components: availability, citizenship, transcripts, dissertation abstract, publication and presentation plan, and information about your Research Advisor co-applicant.
Additional information about the IC Postdoctoral Research Fellowship Program is available on the program website located at: https://orise.orau.gov/icpostdoc/index.html.
If you have questions, send an email to ICPostdoc@orau.org. Please include the reference code for this opportunity in your email.
Research Topic Description, including Problem Statement:
How feasible is the use of synthetic data, in place of rare domain specific data, to train or evaluate ML models? What are the associated risks, benefits and explainability considerations? (I’m using video/imagery systems as an example, but the topic under investigation could cover any physically or digitally collected data or data-sets.)
(Scenario 1 problem statement) It is currently difficult to train novel ML models on data that is sufficiently representative of their final use case, when not much domain specific data is readily available. Specifically: large public data sets tend not to exist for the specific scenarios that are of interest, operational data cannot be made available and/or is insufficient in quantity, production, curation and labelling of ‘real’ data to cover all variants of scenarios can be extremely costly, complex and time-consuming (eg. i-Lids).
(Scenario 2 problem statement) A model pre-trained on publicly or commercially available data has been submitted for evaluation against an operational requirement that represents a similar task. Insufficient domain specific data exists with which to evaluate the capability thoroughly, as it has generated in an uncommon manner.
Questions to be answered:
With advancements in the ability to generate increasingly realistic synthetic data (e.g. by Game Engines in the video example), what is the possibility and utility of generating representative synthetic data to allow training of AI systems used to detect and/or identify specific content, or otherwise enrich live or large operational data feeds?
If we only have access to small amounts of real data with which to test a pre-trained AI, is it possible to synthetically extend the data to a suitably sized test set?
Can the synthetic data be generated with suitable detail to embed the desired information for later extraction?
Are AI’s that have been trained this way subject to any biases in response?
Can the efficacy, accuracy or other performance metrics of AI’s trained on synthetic data be relied upon when the same systems are then given ‘live’ data? And if not, is there a predictable performance adjustment that can be applied?
How does ‘explainability’ work with AI’s trained on synthetically generated data?
What are the ethical considerations around the use of AI/ML systems trained on, or tested against synthetic data?
Example Approaches:
- Scenario 1: Create two ‘real’ datasets and one synthetic dataset, that mimic a true data type of interest. Train two instances of an AI, one on the synthetic and the other on the first real data set. Test both AI instances on the 2nd ‘real’ dataset and compare performance.
- Scenario 2: Make a short ‘real’ dataset. Make a larger ‘real’ data set. Synthetically extend short ‘real’ dataset. Test pre-trained AI to ensure responses are similar to both
NB: ‘real’ as used above, means a dataset that contains true data (non-synthetic) that has been mocked-up or manipulated to provide an accurate representation of operational data.
Key Words: synthetic, training, evaluation, testing, AI, ML
Postdoc Eligibility
- U.S. citizens only
- Ph.D. in a relevant field must be completed before beginning the appointment and within five years of the appointment start date
- Proposal must be associated with an accredited U.S. university, college, or U.S. government laboratory
- Eligible candidates may only receive one award from the IC Postdoctoral Research Fellowship Program
Research Advisor Eligibility
- Must be an employee of an accredited U.S. university, college or U.S. government laboratory
- Are not required to be U.S. citizens
- Citizenship: U.S. Citizen Only
- Degree: Doctoral Degree.
-
Discipline(s):
- Chemistry and Materials Sciences (12 )
- Communications and Graphics Design (3 )
- Computer, Information, and Data Sciences (17 )
- Earth and Geosciences (21 )
- Engineering (27 )
- Environmental and Marine Sciences (14 )
- Life Health and Medical Sciences (45 )
- Mathematics and Statistics (11 )
- Other Non-Science & Engineering (2 )
- Physics (16 )
- Science & Engineering-related (1 )
- Social and Behavioral Sciences (30 )


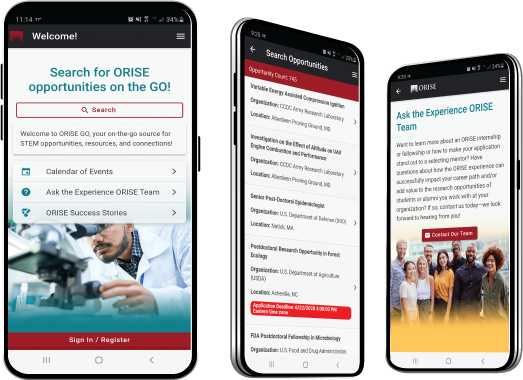
The ORISE GO mobile app helps you stay engaged, connected and informed during your ORISE experience – from application, to offer, through your appointment and even as an ORISE alum!