Robust Multilingual End-to-End Speech Recognition
Create and release your Profile on Zintellect – Postdoctoral applicants must create an account and complete a profile in the on-line application system. Please note: your resume/CV may not exceed 2 pages.
Complete your application – Enter the rest of the information required for the IC Postdoc Program Research Opportunity. The application itself contains detailed instructions for each one of these components: availability, citizenship, transcripts, dissertation abstract, publication and presentation plan, and information about your Research Advisor co-applicant.
Additional information about the IC Postdoctoral Research Fellowship Program is available on the program website located at: https://orise.orau.gov/icpostdoc/index.html.
If you have questions, send an email to ICPostdoc@orau.org. Please include the reference code for this opportunity in your email.
Research Topic Description, including Problem Statement:
Recent deep learning developments have produced dramatic improvements in the performance of speech recognition technologies. Many advancements are due to end-to-end (E2E) neural network models, which optimize a direct mapping from audio data directly to text information with a single network. However, the supervised manner in which these E2E models are trained does not produce state-of-the-art performance results in adverse conditions, such as noisy audio environments and low-resource language scenarios.
With this project, we aim to identify methods to improve E2E speech recognition performance robustness for noisy audio environments and low-resource language scenarios.
Example Approaches:
- Advanced speech enhancement modules could be developed as a data preprocessing step for noisy audio environments. Although using speech enhancement modules to improve the listening quality of noisy audio is a well-established research technique, it is known to be harmful as a speech recognition front-end module. Solving this degradation issue is an active research challenge.
- Pre-training/fine-tuning approaches could be explored for low-resource language scenarios. Supervised E2E models require large amounts of labeled data to achieve state-of-the-art performance results, which is an obstacle for achieving comparable results for low-resource languages. Exploring approaches using self-supervised learning representation models pre-trained with multiple languages and fine-tuning for low-resource target languages is an active research area that could be explored.
Relevance to the Intelligence Community (IC):
Speech recognition is a fundamental Human Language Technology (HLT) capability that provides audio content triage capabilities. New state-of-the-art E2E neural network approaches remove onerous large, labeled data requirements needed to develop a speech recognition capability for a language of interest; however, these gains are at the expense of lower performance results in adverse audio environments and low-resource scenarios. The work described above would identify solutions to these issues which are critical to maintaining a competitive edge in language analysis capabilities.
Postdoc Eligibility
- U.S. citizens only
- Ph.D. in a relevant field must be completed before beginning the appointment and within five years of the application deadline
- Proposal must be associated with an accredited U.S. university, college, or U.S. government laboratory
- Eligible candidates may only receive one award from the IC Postdoctoral Research Fellowship Program
Research Advisor Eligibility
- Must be an employee of an accredited U.S. university, college or U.S. government laboratory
- Are not required to be U.S. citizens
Key Words: #Speech Recognition, #Multilingual, #Deep Learning, #Neural Network, #End-to-end, #Low Resource, #Noise Robustness
- Citizenship: U.S. Citizen Only
- Degree: Doctoral Degree.
-
Discipline(s):
- Chemistry and Materials Sciences (12 )
- Communications and Graphics Design (5 )
- Computer, Information, and Data Sciences (17 )
- Earth and Geosciences (21 )
- Engineering (27 )
- Environmental and Marine Sciences (14 )
- Life Health and Medical Sciences (48 )
- Mathematics and Statistics (11 )
- Other Non-Science & Engineering (2 )
- Physics (16 )
- Science & Engineering-related (1 )
- Social and Behavioral Sciences (29 )


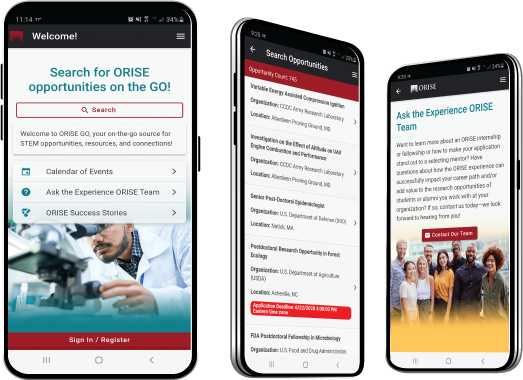
The ORISE GO mobile app helps you stay engaged, connected and informed during your ORISE experience – from application, to offer, through your appointment and even as an ORISE alum!