Air Quality Sensor Data Analytics: What Information Can be Gained From Dense Sensor Networks
A complete application consists of:
-
An application
-
Transcripts – Click here for detailed information about acceptable transcripts
-
A current resume/CV, including academic history, employment history, relevant experiences, and publication list
-
Two educational or professional references
All documents must be in English or include an official English translation.
If you have questions, send an email to EPArpp@orau.org. Please include the reference code for this opportunity in your email.
The research participant selected for this opportunity may collaborate with a multidisciplinary team of EPA scientists devoted to advancing the understanding and use of lower-cost air quality sensors and sensor systems. We have a variety of stakeholders including EPA researchers/policy makers/regulators, regional/tribal/state/local air quality professionals, academics, sensor manufacturers, communities, citizens, etc. Our research efforts provide first-hand knowledge, best practices, techniques, and tools that can inform future sensor use by our stakeholders.
The research participant may collaborate with a multidisciplinary team of EPA scientists devoted to advancing the understanding and use of lower-cost air quality sensors and sensor systems. We have a variety of stakeholders including EPA researchers/policy makers/regulators, regional/tribal/state/local air quality professionals, academics, sensor manufacturers, communities, citizens, etc. Our research efforts provide first-hand knowledge, best practices, techniques, and tools that can inform future sensor use by our stakeholders.
The research participant may conduct research related to a small scale network deployment of lower-cost air quality sensors. The network is part of a remote urban field study being developed in collaboration with a local air quality agency. Data may be used to model the movement of pollutants and to target efforts to reduce local-scale air pollution. The field study design may also allow for the proposal and testing of various network calibration techniques including, but not limited to, reposition-able FEM instruments for collocation. The network deployment may allow for research exploring data processing methods using adjacent nodes or nearby air quality and/or meteorological reference data. Research may involve development of methods for visualizing, animating, and modeling network data and for locating area pollutant sources. Given the large volume of data and measurement artifacts affecting some sensors, the research participant may use programming languages (e.g., R) to analyze data, explore algorithms meant improve data quality, and visualize the data. The research participant may be involved in regular meetings with local air quality professionals and one or more aspects of sensor discovery, planning and implementation of the field deployment, routine monitoring of data from field deployed instruments, and data collection. Commensurate with the level of training, the research participant will have latitude in exercising independent initiative and judgment.
The research participant may also be involved in developing materials to assist in knowledge transfer including peer-reviewed journal articles, reports, best practices documents, presentations, and fact sheets that may be published on the web and/or presented to internal and external audiences. The research participant will have the opportunity to present results at scientific conferences and publish manuscripts. Field deployments may allow the research participant to gain experience in developing the necessary research materials (e.g., quality assurance plans, operating procedures) and to collaborate with regional and local air quality personnel.
This program, administered by ORAU through its contract with the U.S. Department of Energy (DOE) to manage the Oak Ridge Institute for Science and Education (ORISE), was established through an interagency agreement between DOE and EPA. The initial appointment is for one year, but may be renewed upon recommendation of EPA and is contingent on the availability of funds. The participant will receive a monthly stipend commensurate with educational level and experience. Proof of health insurance is required for participation in this program. The appointment is full-time in the Research Triangle Park, North Carolina area. Participants do not become employees of EPA, DOE or the program administrator, and there are no employment-related benefits.
The mentor for this project is Andrea Clements (clements.andrea@epa.gov). The anticipated start date for the appointment is November 1, 2018.
Candidates with a Ph.D. degree from a field that involves quantitative analysis of large data sets such as engineering, atmospheric science, environmental science, computer science, statistics, mathematics, physical sciences, physics, or chemistry would be well positioned to gain from this training opportunity. The degree must be received within five years of the appointment start date. Degrees in Interdisciplinary STEM fields with strong quantitative analysis will also be considered. Individuals whose resume includes field and/or laboratory experience involving air quality monitoring, experience with developing/using/calibrating low-cost sensors and sensor systems, experience in programming for air quality data analysis and/or air quality modeling will be given greater consideration for selection.
Preferred skills include:
- Experience with quantitative analysis of large environmental data sets, air quality data, and/or air quality modeling including experience developing programming scripts to process and review, analyze, and visualize data;
- Experience with large data sets from other fields may also be valuable;
- Experience with spatial mapping, GIS modeling, observational data-driven modeling, source apportionment modeling, or plume modeling may be valuable;
- Direct field and/or laboratory experience in air monitoring, particularly the use of air quality sensors and knowledge of performance metrics, collocation/calibration techniques, and visualization, animation, and modeling of network data;
- The sources of knowledge and experience may include direct experience, academic or direct professional training;
- Skills in R programming is highly preferred although experience with SAS, python, GIS, and/or MATLAB could also be useful.
- Experience giving scientific presentations, authoring peer-reviewed journal publications, and developing other technical documents.
- Degree: Doctoral Degree received within the last 60 month(s).
- Discipline(s):


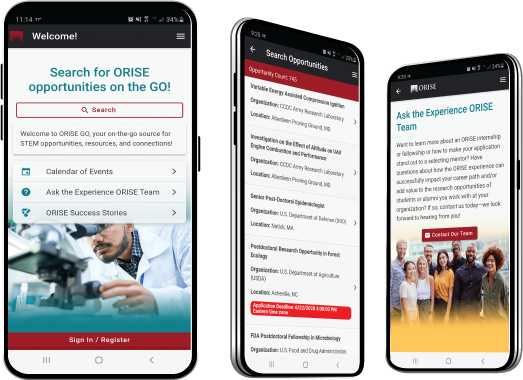
The ORISE GO mobile app helps you stay engaged, connected and informed during your ORISE experience – from application, to offer, through your appointment and even as an ORISE alum!