Innovations in machine learning applications and their implementation to improve our understanding of the planetary boundary layer structure and dynamics
Global climate warming poses a significant challenge to humanity, and despite vast research efforts, the detection and quantification of climate change and its prediction, both in observations and climate models, remains a main focus of the scientific community. Machine learning methods have been utilized exponentially in the past few years to address remote sensing detection and retrieval algorithms, improved climate model parameterizations and even used for climate predictions. They provide the non-linear stochastic functionality needed to describe the Earth Atmosphere complex system such as the cloud system, atmospheric composition and dynamics and their link with the overall radiative budgets that leads to either warming or cooling of various regions over the globe. Among one of the emerging machine learning approaches are weather networks, who work to link different regions based on their similarity in a spatial and temporal (dynamic) sense.
Specifically in this opportunity we are seeking to combine machine learning based methods with weather networks to be able to answer questions that are related to the planetary boundary layer (PBL) structure characteristics under various regimes over the globe, and the link between PBL regimes and other system state variables such as land or sea surface temperature, winds and more. After developing a (multi-layer) weather network that can describe the connections between nodes on a global and regional scales, we will then use available PBL measurement data and network features to develop a prediction scheme for PBL height that will rely on system state in addition to available measurements and that can allow predictions in areas of missing observations using the similarity of PBL structure and dynamic obtained from the weather network construction.
Applicants with a strong computational and machine learning background that are keen to apply their expertise in promoting real-world climate-related questions and uncertainties are encouraged to apply. The advisor, Dr Segal-Rozenhaimer has an expertise in machine learning methods in remote sensing and atmospheric science, aerosol-cloud interactions and radiative budgets.
Location:
Ames Research Center
Moffet Field, California
Field of Science:Earth Science
Advisors:
Michal Segal Rozenhaimer
segalrozenhaimer@baeri.org
(650) 604-4392
- Citizenship: LPR or U.S. Citizen
- Degree: Doctoral Degree.


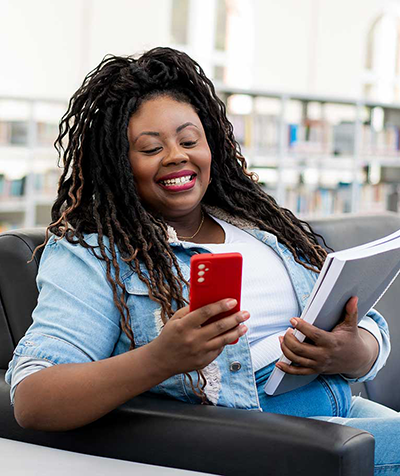
Whether you are just starting your career or already at a senior level, ORAU offers internships, fellowships, research opportunities, and contract positions that can provide you with invaluable experience. Download the ORAU Pathfinder mobile app and find the right opportunity to propel you along your career path!